The Hidden Dangers of AI Stock Photos No One Talks About
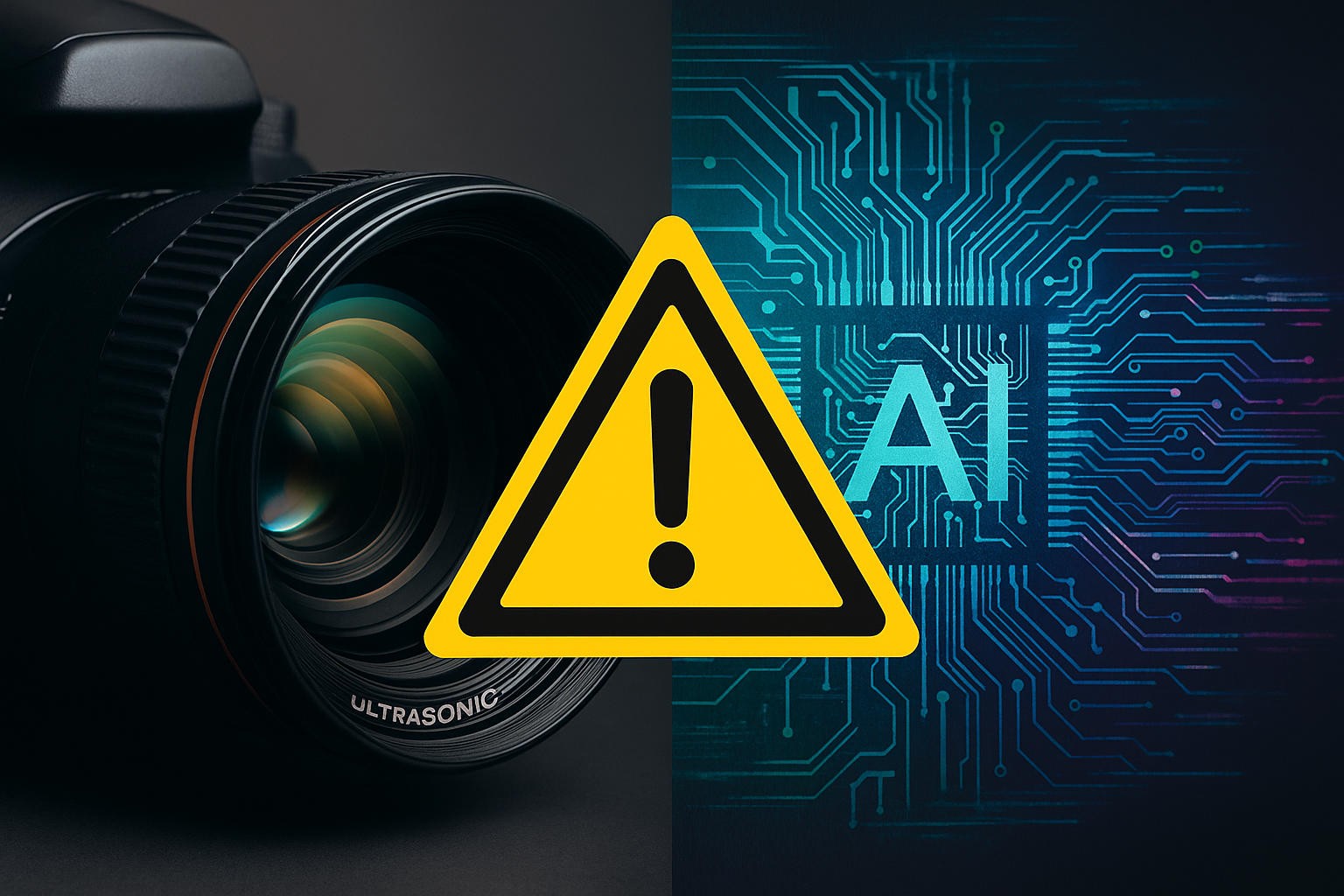
Introduction: The Allure—and What We’re Overlooking
Over just the last couple of years, AI-generated stock photos have gone from quirky novelty to an everyday tool. Platforms like Midjourney, DALL·E, and Stable Diffusion now crank out sleek, high-quality visuals in moments. No more hunting through endless stock catalogs or worrying about model releases and location permits—just type a prompt, and there it is.
But that ease hides serious complications. Legal gray areas, cultural blind spots, hidden costs, and ethical pitfalls lurk behind the clean, clickable surface. In this article, we’ll unpack eight of the biggest risks—from copyright to carbon footprints—so anyone using AI imagery can do so with their eyes wide open. The goal isn’t to scare you off, but to help you wield these powerful tools wisely.
1. Copyright and Legal Hazards
Questionable Training Sources
Most AI art tools learn by scraping the web—ingesting millions (even billions) of images without explicit permission. In January 2023, Getty Images sued Stability AI in the U.K., claiming its Stable Diffusion model was trained on copyrighted photos without a license. A parallel suit in the U.S. echoed the same concerns. That lawsuit is still pending, but it highlights a core issue: if your AI provider relied on unlicensed art, your generated images could carry hidden legal baggage.
Risk of Copycat Content
Even when models train on “clean” data, they can regurgitate outputs that mirror originals too closely. If an image you generate resembles a real photograph—say, the same composition or unique subject—that could trigger a takedown notice or a costly infringement suit. For small teams or solo creators, defending against such claims can erase any savings from skipping traditional stock fees.
A Legal Gray Zone
Copyright law hasn’t yet caught up with generative AI. Courts haven’t definitively ruled on whether AI outputs qualify as “derivative works.” Until clear precedents emerge, every AI-created asset sits in a legal fog. That uncertainty makes using these images a gamble—especially for major campaigns or product packaging.
2. Ethical Gaps and Built-In Bias
Skewed Datasets, Skewed Results
AI mirrors the data it’s fed. If your training set overrepresents Western, light-skinned subjects, the model will underperform on others. Research published in Nature shows many generators deliver more polished results for lighter complexions. Relying solely on AI for a diverse campaign risks unintentionally perpetuating those biases.
Cultural Stereotyping on Autopilot
Type “CEO in office,” and many tools default to a white male in a suit; “nurse at work” often yields a female figure. Those unconscious algorithmic assumptions can slide outdated stereotypes into your brand materials. An underrepresented audience will notice, and it can alienate them faster than any typo.
The Myth of Machine Objectivity
It’s tempting to think “AI is neutral.” In reality, every design choice—what data to include, whose work to exclude, how to label it—bakes human values into the system. With little transparency from providers about their training mix, you may be reinforcing biases you never intended.
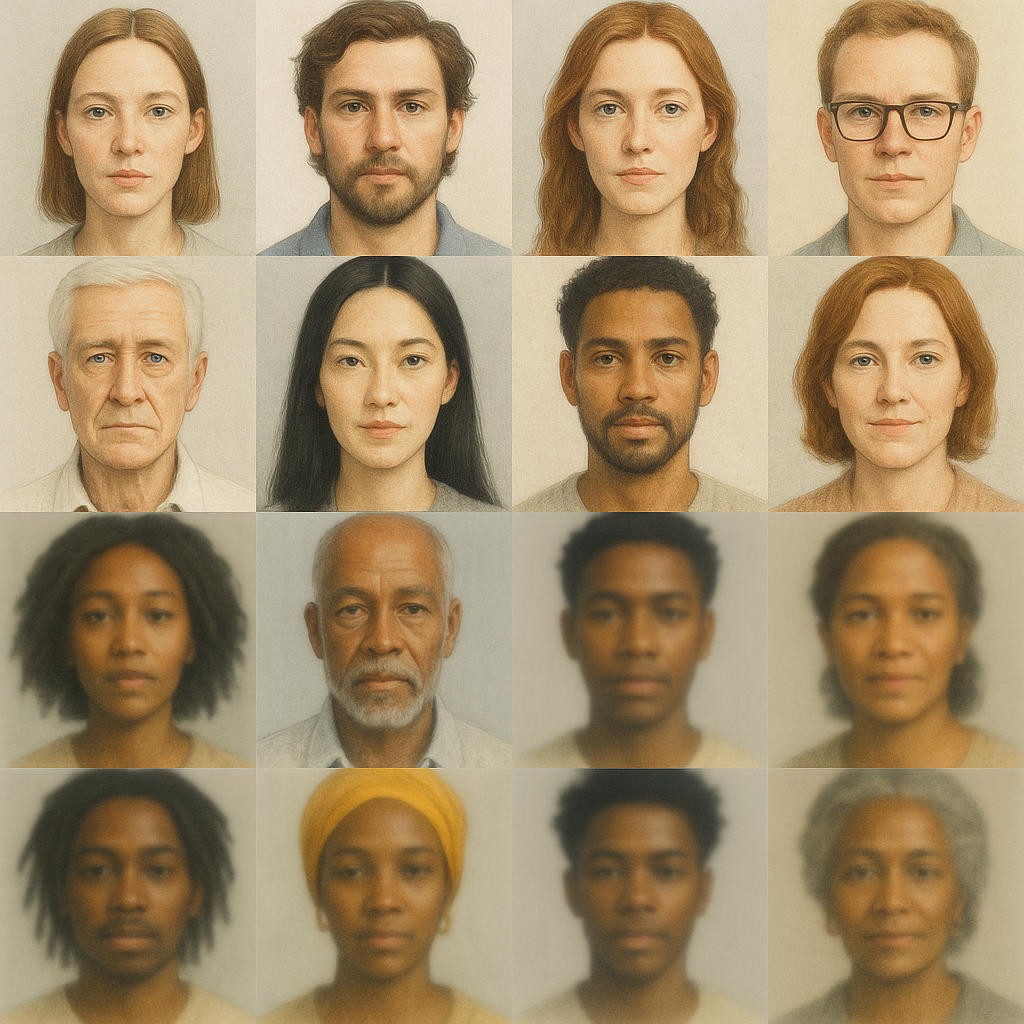
3. Visual Monotony in the Age of AI
When everyone uses the same handful of AI engines, a distinctive “AI look” emerges: flawless skin, perfect symmetry, moody lighting. At first glance, it wows—but over time, it feels cookie-cutter. Your brand runs the risk of blending into a sea of identical hero banners.
Traditional stock libraries, for all their flaws, still offer genuine variety—unique angles, cultural context, accidental magic. AI tends to flatten that richness into a sanitized average. If your story depends on a one-of-a-kind vibe, relying solely on AI can undermine your brand’s distinct voice.
4. Trust and Authenticity at Risk
Backlash When the Truth Comes Out
Picture a nonprofit using AI-generated refugee portraits—only for critics to uncover they’re entirely synthetic. That kind of revelation can shatter donor trust. In sensitive fields like journalism or healthcare, authenticity isn’t optional; it’s the foundation of credibility.
Advertising’s Trust Problem
Getty’s 2023 VisualGPS study reports that 76% of consumers agree “it’s getting to the point where I can’t tell if an image is real,” and 87% say authenticity is very important. When audiences feel misled by AI in your promos, engagement dips—and your reputation takes a hit that no free image can justify.
Trust Is a Long Game
Real photography—even imperfect—often resonates more deeply. A candid laugh or a misty sunrise carries a type of honesty no algorithm can fully replicate. Once trust erodes, rebuilding it costs far more than your initial image budget.
5. Technical and Quality Pitfalls
Despite leaps in realism, AI still flubs basics: extra fingers, nonsensical text on signs, reflections that invent new light sources. Those glitches pull viewers out of the story fast.
And fixing these issues often eats into the promised time savings. Touching up a warped hand or retyping a brand name in Photoshop can take longer than refining a traditional photo.
Worse, platforms update models frequently. The perfect style you love today might vanish with the next release, leaving you scrambling to reproduce past outputs or hunting for archived high-res exports.

6. Environmental Toll of “Virtual” Images
Real-world shoots burn fuel, but AI isn’t carbon-free either. Training a state-of-the-art diffusion model emits CO₂ roughly equivalent to a single trans-Atlantic flight. Serving millions of on-demand renders adds up, too.
Most AI providers host their GPUs in massive data centers and don’t disclose energy sources. If those facilities run on fossil fuels, your “green” virtual shoot may carry a significant hidden carbon cost.
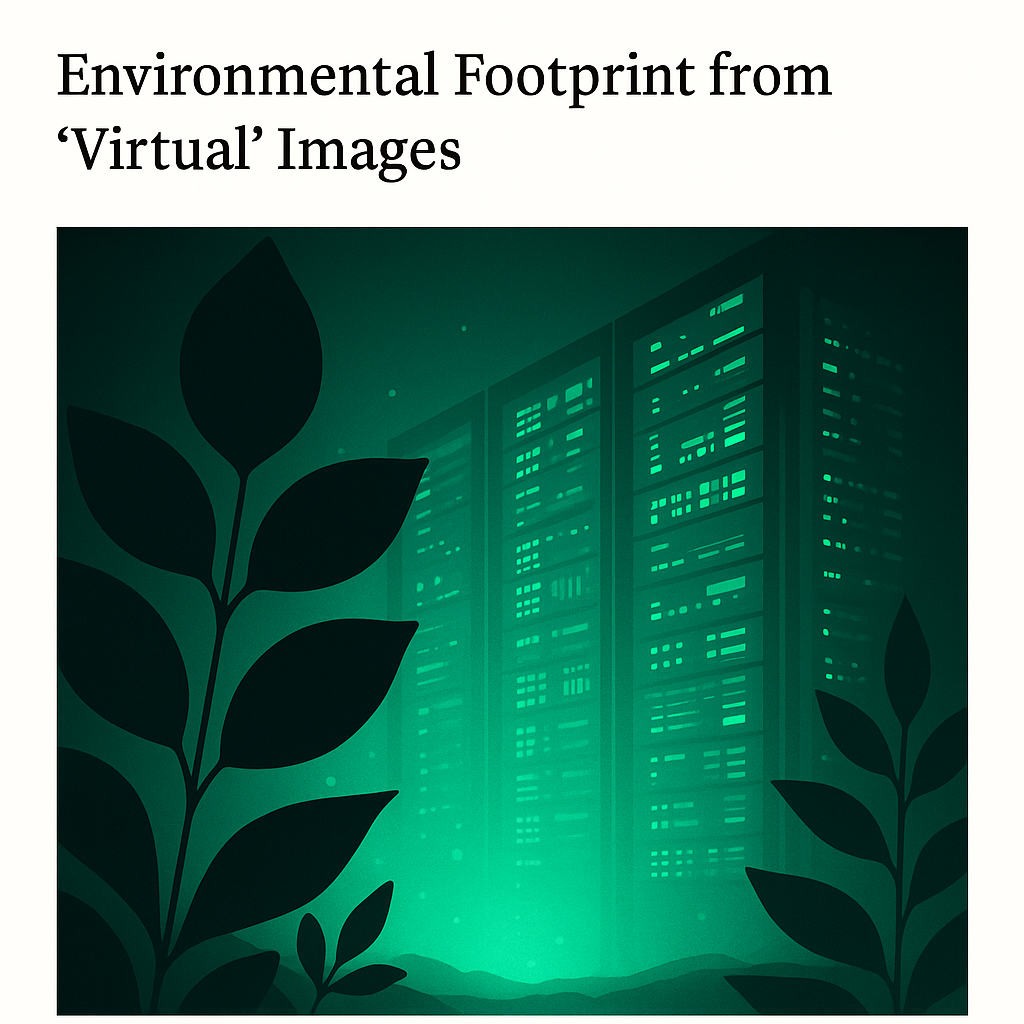
7. Overdependence and Skill Erosion
Photography and design are crafts honed over years—learning to light a scene, frame a shot, retouch by hand. If every junior designer defaults to “type a prompt,” those core skills risk atrophy.
True creative breakthroughs often come from hands-on experimentation: mixing physical media, playing with real light, discovering happy accidents. Overreliance on AI can lull teams into thinking originality is just a few words away—in reality, that muscle needs regular exercise.
As AI outputs become commoditized, the highest value will go to creators who blend analog expertise with digital agility. The rest risk being automated out.
8. How to Spot and Mitigate These Risks
- Audit your licensing: Use platforms with clear, royalty-free terms and archive the TOS at generation time.
- Test for bias: Run sample prompts across demographics and supplement with real photography where AI underperforms.
- Maintain a unique style guide: Develop brand-specific prompt templates and mix in original art to avoid the “generic AI look.”
- Disclose in sensitive contexts: Label AI-generated imagery in journalism, healthcare, or political ads to preserve trust.
- Track your footprint: Ask providers for energy-mix data; consider carbon offsets if your volume is high.
- Keep craft alive: Invest in workshops and cross-train teams on lighting, composition, and manual retouching.
- Archive critical assets: Download high-res exports and save prompt recipes so you can recreate or tweak images if models change.
Conclusion: Proceed with Eyes Wide Open
AI-generated stock imagery offers speed, cost savings, and boundless flexibility. Yet every shortcut carries trade-offs: legal ambiguity, baked-in bias, environmental costs, and potential creative decay. By understanding these hidden dangers, you can adopt AI imagery strategically—using it to amplify your workflow, not replace your judgment.
The most future-ready teams won’t choose “AI vs. photos.” They’ll blend both—leveraging AI for rapid ideation and scalability, and photography for authenticity, emotion, and proof. That balanced approach preserves the human touch while embracing innovation.
Free help with your projects
Need images for your next campaign but don’t have the money to pay for them? Download Free AI Images for personal and commercial use. No licenses, no restrictions.
Comments